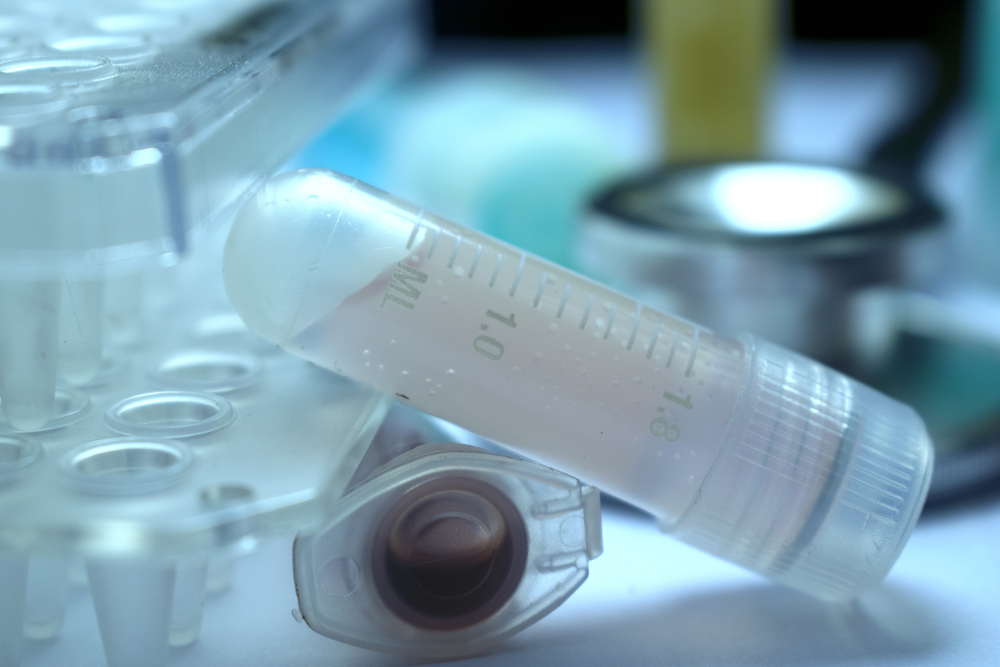
A team of researchers from the Cary Institute of Ecosystem Studies, the University of Georgia, and North Carolina A&T State University have attained a $2 million grant from the National Science Foundation for computer-driven methods of disease forecasting.
The institutions hope to develop tools, spurred by machine-based learning, to predict zoonotic disease outbreaks before they happen. Ebola, Zika, and like-minded pathogens all come from wildlife and livestock. Being able to predict where such pathogens are likely to transfer from animals to people could save countless lives.
“We want to help shift society from a reactive to a proactive approach to managing zoonotic disease,” Barbara Han, disease ecologist at the Cary Institute and study lead, said. “Instead of responding to outbreaks, let’s try to stop them from happening in the first place. Using big data as a potential surveillance tool is an exciting new step toward prevention.”
During the study’s five-year timeline, they will create models based on the various species hosting pathogens, which ones can make the cross-species leap, and what environmental factors allow for that cross-over. These models will take into account a variety of factors, from the physical and life history traits of host species and pathogens to metabolic and reproductive rates, to conditions surrounding already documented disease outbreaks.
“By using data that is global in scale, we are seeking to reveal generalizable features of ‘good’ disease carriers,” Suzanne O’Regan, a researcher from North Carolina A&T, said. “Over 50 life history features are being incorporated into models for most mammal groups.”
They will also consider transmission methods, properties, and pathogen survivability, coupled with a slew of environmental factors such as temperature, precipitation, seasonality, and biome. Scientists will then take all this information and work it into mathematical models to determine how diseases will prosper among animals over time.
“The novelty of this work is in bringing biological realism via machine learning into a classic body of theory, leveraging large sets of biological data available to us,” Han said. “These tools merge data mining and machine learning with established methods of studying disease dynamics to help us think carefully about what’s distinguishing animal groups from each other in terms of zoonotic disease, and eventually, for risk of human spillover and epidemics.”